Author: Kenneth Egwu and Estelle Mbadiwe
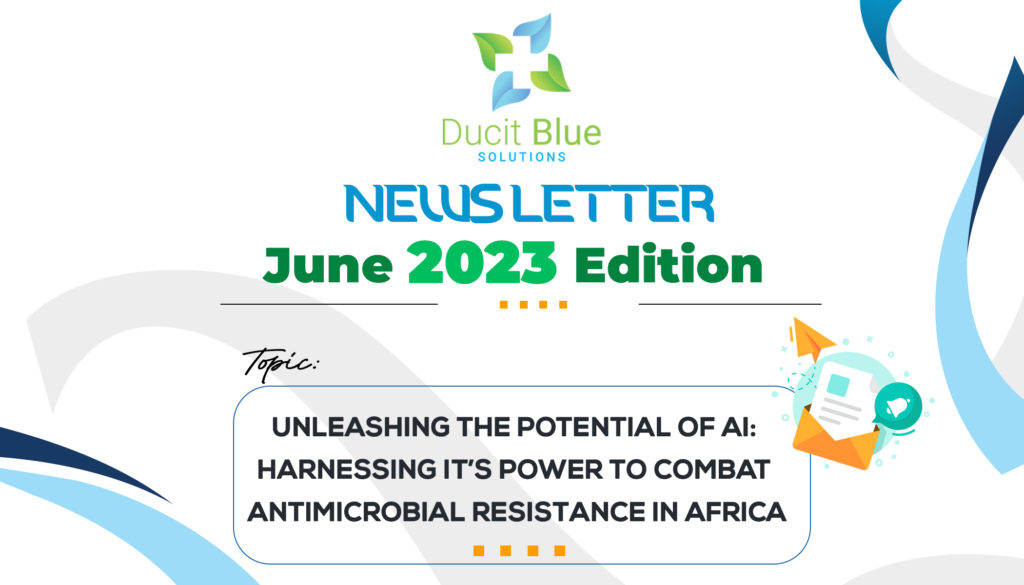
Background
Antimicrobial Resistance (AMR) is seemingly becoming a pandemic, but disproportionately affects people in low-resource settings. In 2019, bacterial AMR was associated with more than 4.95 million deaths ¹. The economic impacts of AMR include lengthened hospital stays, increased healthcare costs, as well as a decline in labour supply and productivity. If the current trend continues, the worldwide economic cost of AMR is predicted to exceed US$ 100 trillion by 2050 ².
Figure 1: Data on the burden of AMR
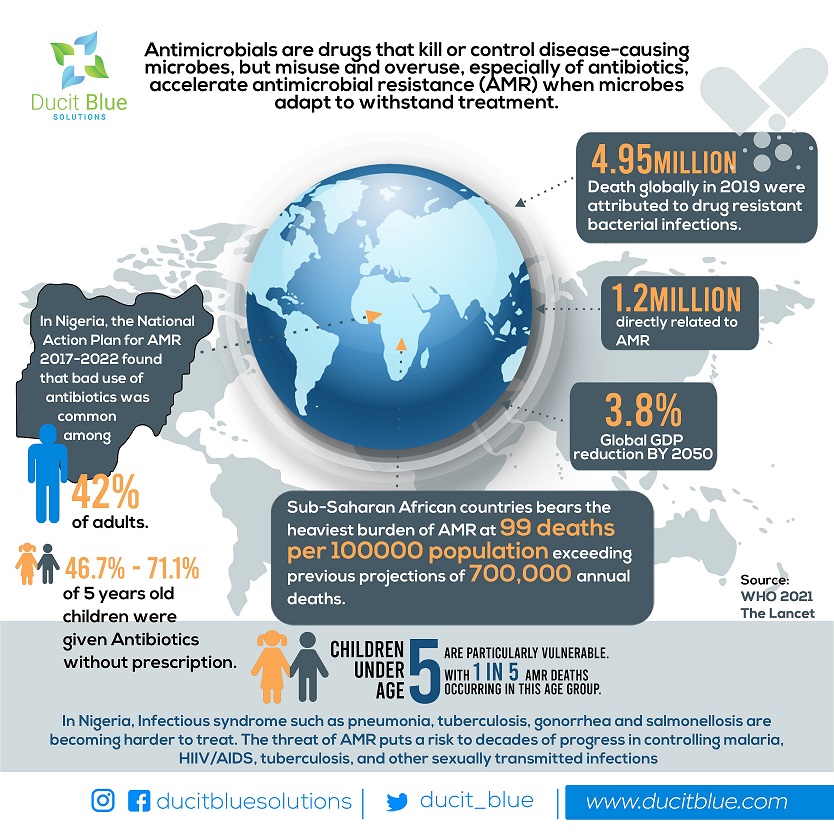
Source: Ducit Blue Solutions Archives
Factors Contributing to the Spread of Antimicrobial Resistance in Africa
AMR poses a threat not only to humans but also to animals, plants, and the environment. Access to quality healthcare services and the eradication of potential infectious agents are requisite in combating the rapid spread of AMR. However, many countries, particularly those with limited healthcare infrastructure, poverty, and poor living conditions, struggle to achieve these goals. For example, in Africa, where 90% of people live in informal houses and lack basic necessities such as sanitation, access to fresh water, and food security, poor living conditions facilitate the spread of diseases ³.
This situation leads to an excessive demand for and dependence on antimicrobials as a means of addressing the problem. The overuse of antimicrobials is a contributing factor to the significant rise of AMR in Africa. Animals are also affected by this cycle of antibiotic abuse, as their owners subject them to irregular doses of antibiotics to increase profitability—a problem that is prevalent in the global food production system.
Moreover, the lack of accurate diagnosis poses a significant challenge to curbing AMR. Arbitrary diagnoses are common in Africa due to the difficulty in accessing standard diagnostic equipment. As a result, inappropriate prescriptions, including the continuous use of antibiotics in cases where they are not necessary, contribute to the trend of resistance. Broad-spectrum antibiotics are frequently prescribed in these situations. Alarmingly, these drugs are also misused by rural communities to manage common viral infections such as the common cold, flu, and even headaches.
Surveillance is a critical issue in effectively intervening and implementing policies to combat AMR in Africa. Real-time data, which are crucial for addressing health-related challenges in society, are often inaccessible in many African countries. Although some estimated data on the prevalence of AMR in Africa exist, there is a need for improvement as these data often do not accurately reflect the actual realities in most settings.
Additionally, the lack of technology in many scientific communities across Africa hinders innovation and research on AMR. While scientific labs in the Global North utilize automated processes, many African labs still rely on analog or manual machines, resulting in cumbersome research outcomes.
Addressing these challenges requires concerted efforts from policymakers, healthcare professionals, researchers, and international organizations. Improving living conditions, promoting responsible use of antimicrobials, enhancing diagnostic capabilities, strengthening surveillance systems, and investing in technological advancements are key steps toward effective eradication of AMR in Africa. By prioritizing these actions, African countries can work towards mitigating the impact of AMR and safeguarding the health and well-being of their populations.
Harnessing the Power of Artificial Intelligence in the Healthcare Industry
The healthcare system is undergoing a remarkable transformative phase with the emergence of Artificial Intelligence (AI), which is bringing significant advancements to precision medicine, drug discovery, and research.
AI can be defined as a machine-based system capable of making predictions, recommendations, or decisions that impact real or virtual environments, based on human-defined objectives 4.
The use of AI in healthcare gained significant recognition in 2016 when AI software integrated into the IBM Watson platform accurately diagnosed a rare form of leukemia in a 60-year-old woman and suggested an effective treatment 5. This breakthrough highlighted the potential of AI in healthcare. It is projected that global investment in Medicinal Artificial Intelligence (MAI) will reach approximately $6.6 billion by 2021, with expectations that AI implementation in healthcare can save up to $150 billion in costs by 2026 6.
Machine learning (ML), a branch of AI, aims to develop algorithms that can learn to predict outcome variables accurately from myriads of collections of predictor variables, which are often not hand-selected and weakly curated. These models are trained using data and then applied to test data to assess their prediction capabilities. The exponential growth of experimental and clinical data, advancements in computational capacity, improvements in algorithm performance, and the increasing need for innovative approaches to combat disease burdens have fuelled interest in the application of ML algorithms to address issues such as AMR over the past few years ⁷. ML modelling approaches have been successfully utilized in various studies to predict pathogen antibiotic sensitivity patterns and detect antimicrobial resistance phenotypes, aiding in the selection of appropriate therapies ⁷.
AI is offering a ray of hope to innovators and healthcare workers in Africa, enabling them to tackle the burdens of AMR and infectious diseases on the continent. Despite facing challenges, AI is gradually making its way into the African ecosystem, showing tremendous potential to enhance the region’s healthcare capacity. This brings new possibilities for Africans to address one of their most pressing challenges—the rapid rise of antimicrobial resistance in the region.
With the utilization of AI, African healthcare professionals and innovators can leverage the available resources to combat AMR effectively. By harnessing the power of AI, Africa can drive advancements in diagnostics, treatment selection, surveillance systems, and healthcare delivery, ultimately improving health outcomes and reducing the burden of infectious diseases in the continent.
Figure 2:
Exploring the Mechanisms of Machine/Deep Learning Processes
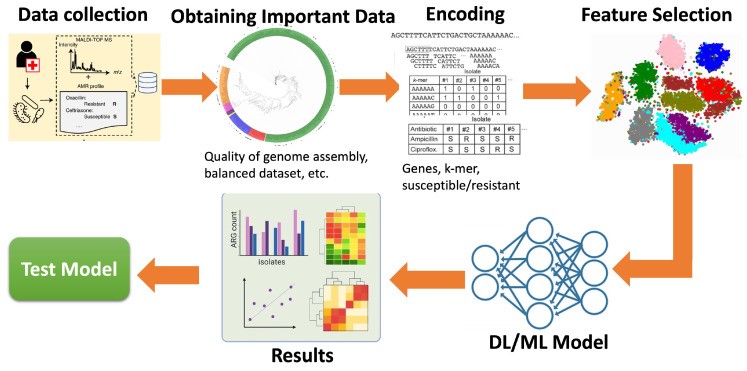
Source: Tabish Ali et al
Leveraging AI for Combatting Antimicrobial Resistance (AMR): Some Applications
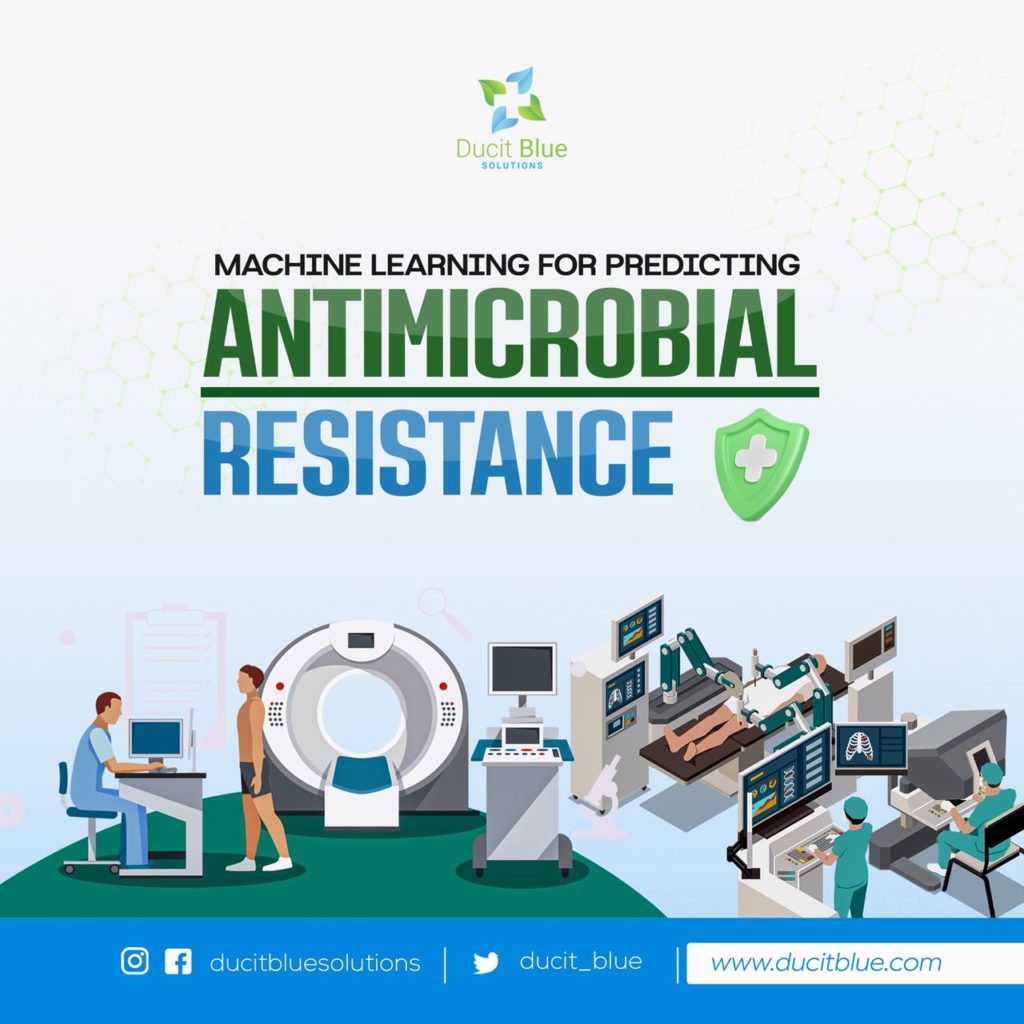
The World Health Organisation listed antibiotic resistance as one of the top ten global public health risks confronting humanity, and artificial intelligence is proving to be a powerful weapon in the fight against it ⁸. Numerous AI tools has emerged in the recent years and they are reshaping our daily activities and facilitating the ease of research, education and drug discovery.
Figure 3: Applications of AI in AMR
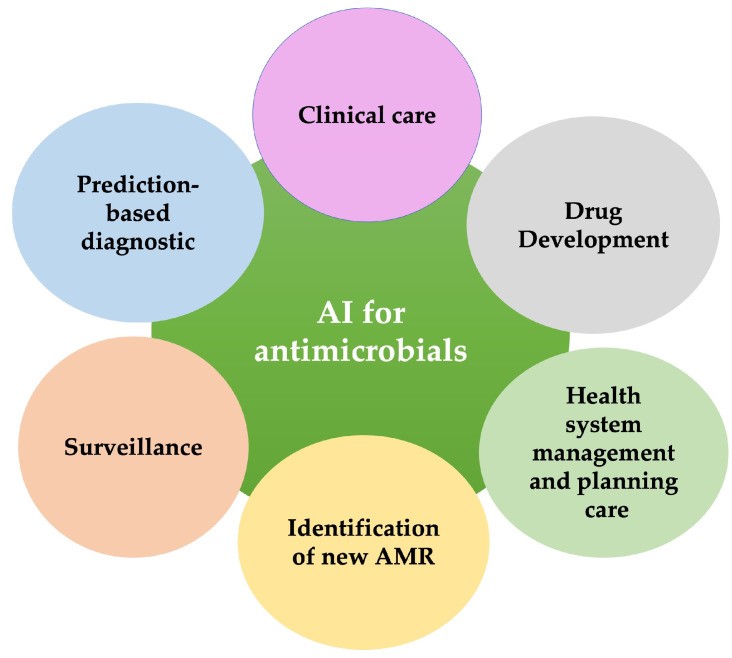
Source: Tabish Ali et al
AI can be useful in fighting AMR in the following ways;
Drug discovery: The potential for AI has no bounds and is becoming more realistic as new models keep emerging. Drug discovery is a tedious and often complicated process costing millions of dollars, and even more difficult for researchers in Africa where access to good laboratories is often difficult. An antibiotic molecule that can kill Acinetobacter baumannii, one of the most hazardous antibiotic-resistant bacteria, was found by Canadian and US scientists using “deep learning” ⁹. A. baumannii, which is notoriously challenging to eliminate, infects hospital patients who are already at risk for infection, such as premature infants and those with weakened immune systems ⁹. Discovery of new antibiotics, especially ones effective against resistant pathogens could mean a step forward in overcoming AMR. The AI technology can evaluate the biological performances of potential drug candidates using a virtual environment, looking at how they could interact with real patients and how effective they might be at treating specific diseases.
Precision medicine: The aim of precision medicine is to ensure that the appropriate patient receives the appropriate care at the appropriate time. AI can deliver precision medicine for the detection and treatment of infectious diseases in Africa. For instance, hemozoin detection for malaria using nuclear magnetic resonance (NMR) offers the ability to identify the development of parasite medication resistance. Another illustration is for tuberculosis, where AI could be used to quickly identify drug resistance, ascertain host immunity, personalise drug therapy, and forecast relapse-free cure ¹⁰.
Improve diagnosis and treatment outcome: Standard diagnostic methods are usually time consuming and prompt the use of broad-spectrum antibiotics, which affects the gut microbiomes and foster infections like C. difficile. Through the use of flowcytometer antimicrobial susceptibility testing and supervised machine learning, several studies have used AI to reduce the diagnostic time to as little as three hours ¹¹. Support Vector Machine (SVM) and Set Covering Machine (SCM) models have been used to learn and predict the resistance Tetracycline, Ampicillin, Sulfisoxazole, Trimethoprim, and Enrofloxacin against Actinobacillus pleuropneumoniae of which both models showed an accuracy of more 90% ¹².
Improved adherence to medication and Infection Prevention and Control protocols: Lack of compliance with infection prevention and control guidelines exacerbates the issues of inappropriate antibiotic use and antimicrobial resistance. Poor adoption of IPC measures is a common cause of hospital acquired infections (HAI) which increases the chances of mortality, morbidity and economic loss ⁴. The recent years have seen numerous AI inspired applications that reminds patients of their medications and contains necessary details on how to adhere to IPC measures. It is possible to convey accurate and current information concerning antibiotic resistance via AI-powered chatbots and virtual assistants. These resources are capable of interacting with the general public, responding to inquiries, and offering advice on proper antibiotic usage, infection prevention, and the significance of finishing treatment courses. AI can also make it easier to communicate in multiple languages with different populations in Africa.
Surveillance: Modern AI surveillance tools are able to analyse large amounts of data, monitor, and track antibiotics supply and consumption using amazingly accurate classifications. AI has a key role to play in building effective AMR surveillance networks. AI systems can identify trends and early warning signals of AMR epidemics by analysing a massive quantity of data, including clinical records, test results, and prescription patterns. Researchers and policy makers can utilize these data to make informed decisions.
Advancement in AI Adoption within the African Healthcare System
Artificial intelligence is playing a significant role in understanding the complexity of AMR globally and Africa is not left out. MAI was historically piloted in Africa in the middle of the 1980s. The deployment of MAI in Kenya improved the quality of the interactions between health workers and patients. Similar to this, in the Gambia, rural health personnel were helped to recognise life-threatening diseases in outpatient clinics by a probabilistic decision-making system. The MAI detected 88% of instances, which was a respectable performance. Based on a cost-and-effectiveness methodology, nurses in South Africa also prescribed drugs using Computerised Aid to Treat (CATT) ¹³.
AI application in Nigeria has also improved the pharmaceutical industry. Five high school students created an app to detect fake drugs in Nigeria based on MIT open-source software. Their brilliant idea gained recognition after winning a Silicon Valley competition in 2018. Also, a Nigerian-trained chemist, received the grand prize of the Hello Tomorrow Global Challenge in 2019 for his AI-hyperspectral platform for authenticating pharmaceuticals ¹⁴, ¹⁵.
The Council for Science and Industrial Research (CSIR) in South Africa deployed an expert AI system to pinpoint the favourable environmental circumstances that will trigger cholera epidemics. The system relied on gathering historical data and expert knowledge, but it also combined meteorological and biophysical variables with epidemiological data to create a hazy surface of outbreak risk potential ¹⁶. Also, a population-based diabetic retinopathy screening programme in Zambia’s Copperbelt area used a deep learning artificial intelligence model made up of the combination of two convolutional neural networks to find referable vision-threatening diabetic retinopathy ¹⁷. The study demonstrated the clinical efficacy of the AI model in identifying referable diabetic retinopathy, vision-threatening diabetic retinopathy, and diabetic macular oedema in a real-world diabetic retinopathy screening programme in Zambia, where the retinal images were primarily taken by non-medically trained technicians.
Furthermore, Viebeg Technologies, a venture capital-backed health technology business, is assisting in enhancing access to affordable healthcare by assisting healthcare facilities in Central and East Africa with real-time supply procurement. It makes use of artificial intelligence (AI) to control the supply chain activities (from shipping to warehousing, distribution, and inventory management) and make sure that healthcare institutions have the precise medical supplies in store ¹⁸.
Furthermore, Artificial intelligence is used by the Nigerian firm Aajoh to assist patients who transmit a list of their symptoms via text, audio, and photos in diagnosing their medical condition ¹⁹. Also, the Kenyan digital health data platform AfyaRekod enables medical facilities to collect, store, have real-time access to patients’ health data. The platform makes smart, data-driven judgements using AI and numerous blockchain modules, enabling doctors to give patients better treatment ¹⁹.
Navigating AI Challenges in Africa: Overcoming Barriers to Adoption and Implementation
The global application of AI in the healthcare system faces various challenges, including the high costs associated with establishment, maintenance, and technical expertise. However, these challenges are further pronounced in Africa due to additional factors such as poor governance and healthcare funding. Inadequate infrastructure, exemplified by low Internet penetration (39%) and sociocultural barriers, significantly hinder the development of AI in Africa 20. The lack of access to electricity across the continent poses a major obstacle to implementing and sustaining digital techniques, including in the healthcare sector, as approximately half of Africans live without electricity. Moreover, the high costs involved in hardware acquisition, computing resources, and maintenance further impede the successful implementation of AI in Africa.
Another significant hurdle is the limited availability of high-quality data for training AI models. The accuracy of forecasts depends on the quality of the underlying data, and the scarcity of comprehensive and representative data poses a challenge. It is crucial for fair algorithms to consider all racial groups and ensure unbiased outcomes. Additionally, the application of AI requires access to electricity, technology, and the development of healthcare professionals’ capabilities 21.
Lack of Standardisation and interoperability also limit the use of AI. For efficient use of AI in the healthcare system, standardisation of data formats, vocabularies, and protocols is essential. Global standards and interoperability frameworks are, however, lacking for the exchange of AMR-related data. In addition, it is essential to validate AI effectiveness and dependability in the context of AMR for AI models to be adopted and accepted. AI models’ interpretability and lack of conventional validation procedures may prevent their full integration into clinical practise.
Addressing these challenges requires collaborative efforts from various stakeholders. Governments, international organizations, and private sector entities need to invest in improving infrastructure, expanding electricity access, and reducing the cost barriers associated with AI implementation. Enhancing data collection and quality standards, while promoting inclusivity and fairness in algorithm development, are also essential. Additionally, capacity-building programs and training initiatives should be prioritized to equip healthcare professionals with the necessary skills to effectively leverage AI technologies.
By overcoming these challenges, Africa can unlock the full potential of AI in healthcare, leading to improved healthcare delivery, enhanced diagnostics, and better health outcomes for its population
1. Improve Infrastructure: African countries need investments in technology infrastructure, capacity building programs, and partnerships to overcome financial limitations and the shortage of skilled professionals trained in AI and data analytics. Addressing the lack of reliable electricity is crucial. Encouraging alternative means of power generation, such as solar energy, can help ensure uninterrupted access to electricity. Additionally, efforts should be made to enhance network speed and connectivity, enabling the smooth operation of AI tools and systems.
2. Training and Capacity Building: Healthcare workers need to be effectively trained to understand how AI operates and how to utilize AI tools in the healthcare system. Allocating sufficient funds and resources to strengthen the capacity of African professionals in AI is essential. Incorporating AI education into the undergraduate curriculum, especially for health students, can help prepare these next generation of healthcare professionals to effectively leverage AI technologies.
3. Data Quality and Research: African researchers play a crucial role in providing quality data for accurate predictions using AI models. Data availability and quality are essential for successful AI implementation. Efforts should be directed towards improving data infrastructure, promoting data sharing collaborations, and ensuring data accuracy and completeness. This will enable AI algorithms to analyze diverse datasets and provide valuable insights into AMR trends and patterns Governments, international organizations, and individuals should inspire and support research initiatives through funding and the provision of resources and modernized laboratory equipment. This will enable researchers to generate high-quality data that can contribute to advancements in AI applications for healthcare in Africa.
4. Collaboration and Partnerships: Encouraging collaboration among stakeholders is vital for the successful implementation and advancement of AI in healthcare. Governments, academic institutions, private sector entities, and international organizations should collaborate to establish partnerships that facilitate knowledge sharing, resource allocation, and the exchange of best practices. By working together, they can drive innovation and accelerate the adoption of AI solutions for combating antimicrobial resistance and improving healthcare outcomes in Africa.
5. Equitable Access: Ensuring equitable access to AI innovations is essential to avoid exacerbating healthcare disparities. Efforts should be made to overcome barriers such as affordability, infrastructure accessibility, and language diversity, making AI solutions accessible to all segments of the population.
6. Localization of AI: Contextual relevance is another critical aspect. Solutions developed for AMR in other regions may not directly apply to the African context due to variations in healthcare systems, socioeconomic factors, and resistance patterns. Therefore, it is crucial to customize AI models and algorithms to align with the unique needs and circumstances of African countries.
By implementing these recommendations, Africa can create an enabling environment for the effective utilization of AI in healthcare. This will not only address the challenges faced but also unlock the full potential of AI to revolutionize healthcare delivery, enhance diagnostics, and improve health outcomes for the African population.
In conclusion, AI innovation holds tremendous potential for addressing AMR in Africa. By harnessing the power of AI, African countries can make significant progress in combating this global health challenge. Moreover, ethical and regulatory considerations should guide AI innovation for AMR. Establishing robust ethical guidelines and regulatory frameworks specific to AI in healthcare and AMR will ensure patient privacy, data protection, and fairness in algorithmic decision-making. In the same vein, collaborative partnerships among policymakers, healthcare professionals, researchers, technology developers, and international organizations are vital. Knowledge sharing, interdisciplinary approaches, and resource pooling will facilitate the development, implementation, and sustainability of AI innovations for AMR in Africa.
By addressing these challenges and embracing AI innovation, Africa can make significant strides in combatting antimicrobial resistance. This will not only protect the health and well-being of its population but also contribute to the global efforts in mitigating the threat of AMR.
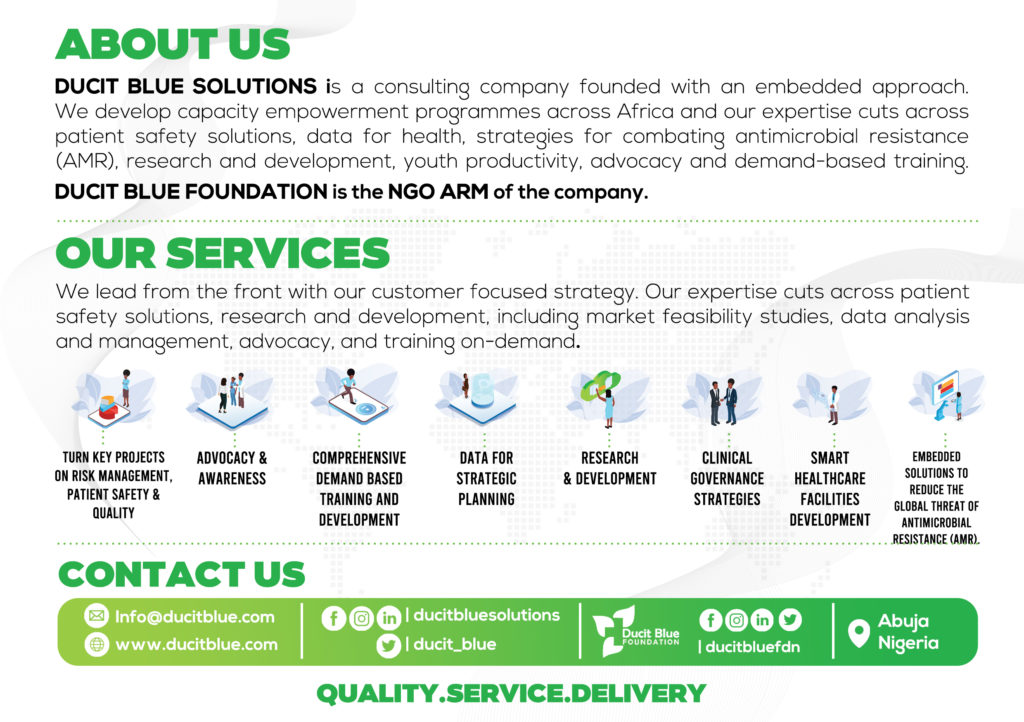